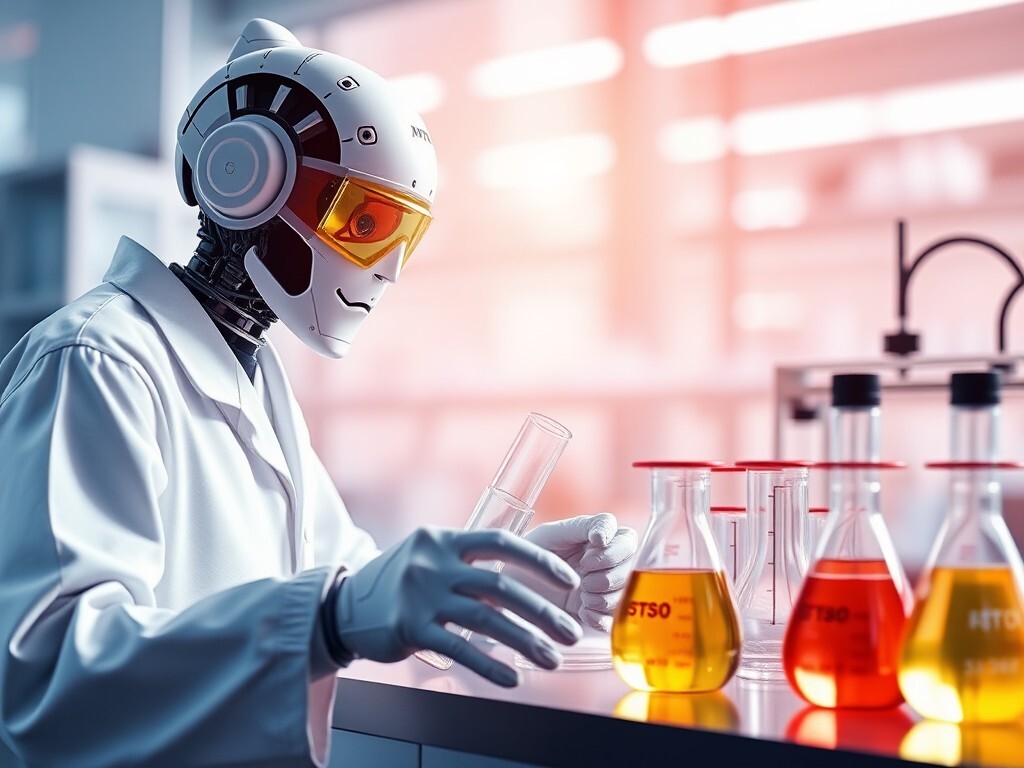
The global COVID-19 pandemic, caused by the novel coronavirus SARS-CoV-2, has had far-reaching consequences, with more than one hundred and forty-eight million infections and approximately two million deaths (da Silva Torres et al., 2022), leaving a lasting impact on public health and economies worldwide. Despite extensive research efforts, the rapid mutation of the virus and its novelty pose significant challenges for the development of effective treatments and vaccines. While some drugs such as Paxlovid and Remdesivir have been approved for emergency use, the search for more targeted and potent small-molecule drugs continues to be a priority. Enter Medgnosis’ GenAI system, an innovative platform designed to accelerate the discovery of novel small molecules that can effectively inhibit key viral proteins.
The Quest for Effective Small Molecules
One of the critical challenges in fighting COVID-19 is targeting the essential structural proteins of the virus, which are responsible for viral replication and infection processes. These include the spike glycoprotein, replicase polyprotein 1a, and replicase polyprotein 1ab. These proteins are vital in the infection cycle, with the spike protein being central to the entry of the virus into host cells (Li et al., 2024; da Silva Torres et al., 2022; Shang et al., 2020).
Medgnosis has focused its AI-driven drug discovery efforts on these proteins, generating forty-seven novel small molecules using its proprietary GenAI platform. These molecules were tested in silico (computer simulations) for their binding affinities and compared with existing drugs such as Paxlovid and Remdesivir. The results were promising, with several Medgnosis molecules showing higher binding affinities, indicating their potential to inhibit SARS-CoV-2 more effectively than current treatments.
How the GenAI Platform Works
The GenAI system leverages artificial intelligence to explore vast chemical spaces and to generate novel molecules. By analyzing the structures of specific viral proteins and predicting their interactions with potential drug candidates, this platform accelerates the time-consuming process of drug discovery. In the case of COVID-19, the GenAI platform was able to rapidly generate hundreds of small molecules, targeting the spike and replicase proteins, which are crucial for viral replication and infection.
“What sets Medgnosis’ GenAI apart is its ability to generate molecules that exhibit favorable drug-like properties, such as compliance with Lipinski’s rule (a set of criteria for drug-likeness), high binding affinity, and excellent bioavailability amongst other properties.” said Joshua Yentumi, co-research lead, Medgnosis. These characteristics make the molecules not only promising in terms of efficacy, but also suitable for development into actual drug therapies.
A detailed technical explanation of the inner workings of the system has been fashioned into a research paper “LAIgnd: Revolutionizing Drug Discovery with Advanced AI-Driven Molecule Generation” which is currently under review for publication.
Comparing Novel Molecules to Existing Drugs
Medgnosis tested its AI-generated molecules against Paxlovid and Remdesivir (images 2 and 3 below), two drugs currently used to treat COVID-19. Remarkably, several of the molecules generated by GenAI outperformed the established treatments in terms of binding affinity and other drug-likeness metrics. Binding affinity is a critical measure of how effectively a drug can bind to its target protein, with higher affinity often correlating with a stronger inhibition of viral activity.
One standout molecule, designed to target the spike glycoprotein (image 4), has demonstrated favorable drug-like properties. With its high binding affinity and good blood-brain barrier (BBB) permeability score, this molecule shows promise as an antiviral agent and has potential applications in central nervous system (CNS) therapies. Additionally, it has a high bioavailability score, making it suitable for oral administration (image 1 below). To view the full report on this compound and their performance, visit: https://medgnosis.ai/P0DTC2_336_report.html
Image 1: Medgnosis hit molecule characteristics on COVID-19 Spike protein. [Access the full image here| full report sheet here].
Image 2: Comparison between Medgnosis’ generated molecules and existing COVID-19 drugs [Access the full image on Replicase Polyprotein 1ab here | full report sheet here | full performance here]
Image 3: Molecule comparison between Medgnosis’ molecules and existing COVID-19 replicase polyprotein 1a drugs. [Access the full image on Replicase Polyprotein 1a here | full report sheet here | full performance here]
A B
Image 4: A: Ball-and-stick representation of hit molecule generated against the SARS-CoV-2 spike protein. B: 2D representation of hit molecule generated against SARS-CoV-2 spike protein
Pharmacokinetic Insights into Spike Glycoprotein Lead Molecule
The novel coronavirus pandemic has had a significant impact on global health care. The healthcare industry has achieved significant results in the development of small-molecule drugs and vaccines that contribute to curtailing the spread and casualty rate of the virus; however, more work needs to be done in the area of developing even more effective medications against viral proteins that exacerbate the infection. To this end, artificial intelligence can significantly improve the rate of discovery of new drugs for such targets. Absorption, Distribution, Metabolism, Excretion, and Toxicity (ADMET) are critical properties for evaluating the suitability of potential compounds as drug molecules (Li et al., 2023). Experimentally evaluating these properties to appropriately select and optimize lead molecules can be a costly and labor-intensive endeavor.
Several machine learning-based tools, such as ADMETlab (Dong et al., 2018), admetSAR (Cheng et al., 2012), and Pharmaco Kinetics Knowledge Base (PKKB) (Cao et al., 2012), have been developed to accurately determine these properties computationally, providing a baseline from which in vivo studies can proceed.
Our GenAI pipeline employs a machine learning-based ADMET property prediction tool called ADMETAi®. This is a fast and accurate ADMET property prediction tool that uses a graph neural network to make predictions (Swanson et al., 2024).
In determining the physicochemical properties of the molecule, the results suggested that the molecule had an optimal molecular weight that satisfied the Lipinski rule of five, which states that oral drugs should have a molecular weight of less than 500 Da for optimal bioavailability. At 292.379 Da, this compound was well within the desirable range, potentially facilitating good oral absorption and blood-brain barrier penetration. Additionally, the molecule had an octanol-water partition coefficient (logP) value of approximately 1.7, demonstrating a good balance between aqueous solubility and membrane permeability. The logP coefficient measures the lipophilicity of a molecule, with a value range generally accepted to be less than 5. Approximately ninety (90%) percent of the drugs developed for human consumption are absorbed by the small intestine (Azman et al., 2022); thus, drug permeability across this membrane is one of the most important pharmacokinetic properties of a good molecule. The Topological Polar Surface Area (TPSA), measured in Ų, determines the likelihood of a potential molecule crossing the intestinal barrier. Values <140 Ų are associated with good intestinal absorption, whereas values <90 Ų are favorable for BBB penetration. At 78.76 Ų, our lead compound is predicted to have good absorption properties and potential CNS penetration.
Drug-likeness is crucial for evaluating compounds for drug development, as it helps identify potential failures early, improving success rates and reducing costs.(B. Li et al., 2024). Using the Quantitative Estimation of Drug-likeness (QED) score, one can immediately determine whether a molecule could be a drug. Ranging from 0 to 1, with higher values indicating greater drug-likeness, the lead molecule obtained a value of 0.7124, suggesting that this compound has good overall drug-like properties.
The Ames test uses bacterial strains such as Salmonella typhimurium to assess the mutagenic properties of chemicals by monitoring reverse mutations. This is a preliminary screening tool for evaluating the carcinogenic potential of chemicals or drug-like molecules (Uesawa, 2024). Lower values indicate a lower mutagenic potential. A score of 0.3024 combined with a low carcinogenicity and moderate clinical toxicity score of 0.0897 and 0.3518, respectively, suggests a relatively low mutagenic and carcinogenic risk, together with a low risk of toxicity in a clinical setting of the lead compound.
This molecular profile presents a promising hit compound with well-balanced physicochemical properties and favorable predicted characteristics. The compound demonstrated good drug-likeness scores, low toxicity risks, and promising bioavailability. Its moderate synthetic accessibility, which measures the ease of synthesizing a compound, suggests a balance between novelty and the feasibility of production.
The Road Ahead: From AI to Real-World Application
Although the results of these in silico tests are promising, further laboratory validation is required before these molecules can progress to clinical trials. However, the rapid generation and testing of drug candidates using AI marks a significant advancement in drug discovery, particularly in response to rapidly evolving viral threats, such as SARS-CoV-2.
The Medgnosis GenAI platform represents an important step forward in the fight against pandemics. By accelerating the development of small molecules targeting specific viral proteins, the platform has the potential to revolutionize the way we approach drug discovery in the future. As co-research lead Mbatuegwu David explains, “Our AI system is designed to generate novel molecules and predict their potential interactions with disease proteins. By rapidly exploring vast chemical spaces, we aim to accelerate the discovery of promising drug candidates and reduce the time it takes to bring new treatments to market.”
“In a world where time is of essence, particularly amid a pandemic, the Medgnosis GenAI platform offers a glimmer of hope. By generating small molecules that outperform existing treatments like Paxlovid and Remdesivir, the system demonstrates the power of artificial intelligence in the battle against COVID-19 and future viral diseases.” said Obi Ebuka David, lead researcher, Medgnosis.
While further testing is necessary, the success of GenAI in generating promising drug candidates marks a significant milestone in the journey towards more effective treatments for SARS-CoV-2 and beyond.
A Call to Action
As Medgnosis continues to break new ground in AI-driven drug discovery, the opportunity to collaborate with us is now more critical than ever. Our cutting-edge GenAI platform is not only accelerating the discovery of treatments for COVID-19 but also revolutionizing the future of pharmaceutical innovation. For healthcare leaders, researchers, and investors looking to stay ahead in the fight against pandemics, Medgnosis offers a unique and powerful solution.
Don’t miss out on the chance to be part of this groundbreaking journey. To explore our latest research and learn how our AI technology can support your mission in advancing healthcare, visit medgnosis.ai. Join us in pushing the boundaries of science and technology to shape the next era of global health solutions.